Cost-Manufacturability Optimization
Reducing Cost, Improving Endurance and Performance of an Imaging System
A common challenge for companies is the endless search to optimize their performance and reduce costs in the fastest and most efficient way possible. That was the case for a company specializing in imaging and security systems, whose target product for optimization was a hyperspectral surveillance system (HSS) featuring night-vision/thermal-vision features (SWIR and LWIR) imaging capabilities).
To achieve their design goals, the team turned to Parallel Pipes’ artificial intelligence driven generative design platform (AI-GDP). To this end the design goals submitted to the platform in order of importance were: cost-to-manufacture, optimal image quality, and durability. Practically this meant lowering cost and improving performance were weighted higher than any other factors. System durability was also included as design goal, because the system had to function reliably for extended usage. With Parallel Pipes’ platform the design was automatically improved over multiple iterations, considering the boundary conditions provided. The final optimized design was obtained by the team, and included an optimal combination of standardized components and generative design-modified geometry.
This led to the company to save more than 3 months of estimated development time, reduce manufacturing costs by more than 60% compared to the first design, and save an estimated $80,000 on development costs. The final design obtained was used by the company to carry out the tests prior to the launch of the product to the market.
Challenge
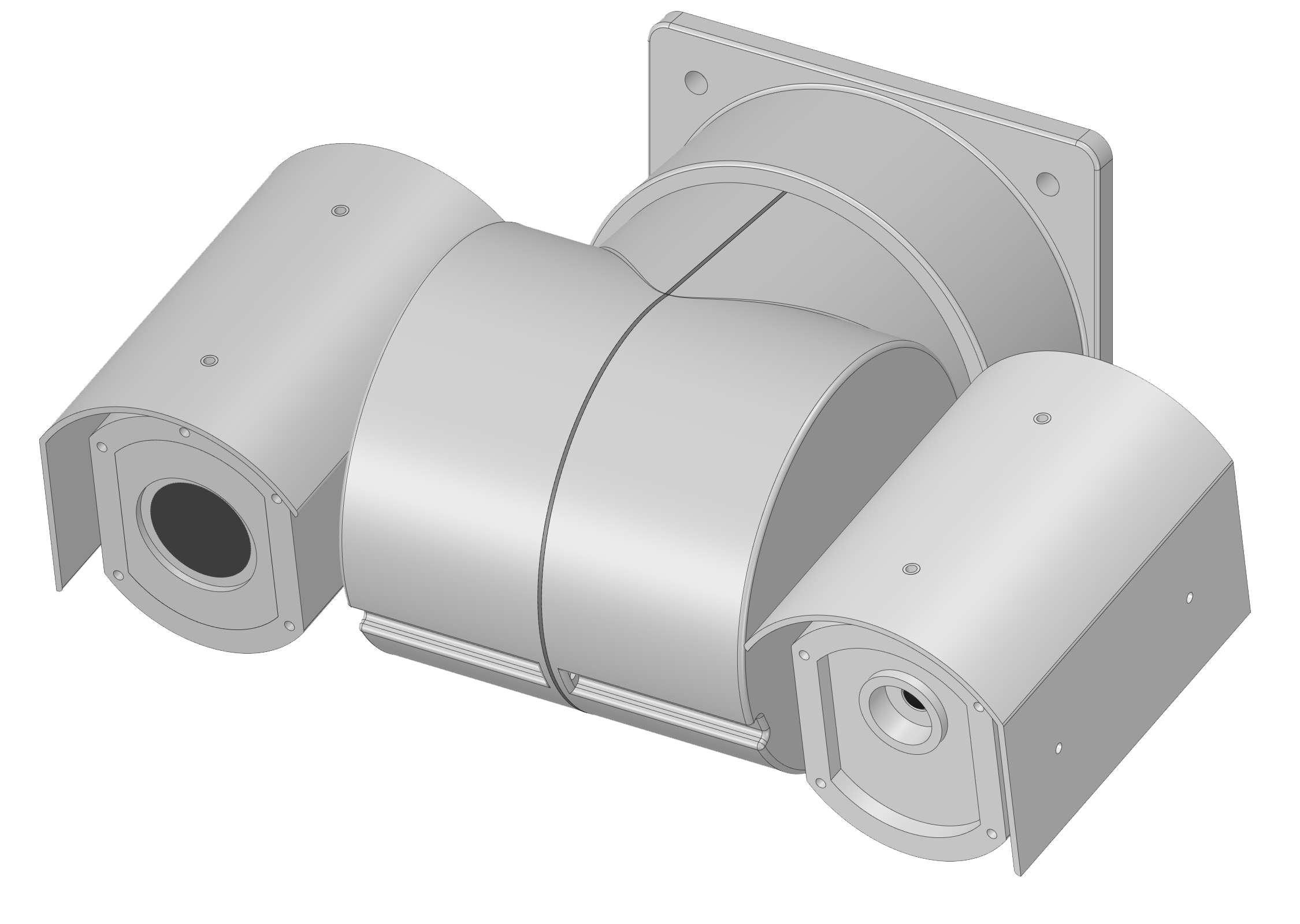
(original design, Human-generated)
Traditional CAE tools for security systems involve staff training and months of work before reaching positive outcomes due to their complexity, which require years of experience in order to use effectively, not to mention cost. AI and machine learning are useful in design optimization for exploring better solutions in a limited time [1]. With Parallel Pipes’ design-to-manufacture approach, an ability to optimize over both optics and robotics components, the team decided to use Parallel Pipes, aiming to save time and production cost by delegating the project to Parallel Pipes’ AI-GDP.
To begin, they uploaded an initial design to the platform. Their initial design (shown in “Figure 1”) met the durability and performance requirements.
Nonetheless, the detection range of the shortwave infrared (SWIR) camera was only 30 meters with adequate quality, and the longwave infrared (LWIR) image had noise regardless of range. In addition the entire system was expensive to manufacture.
Design Goals
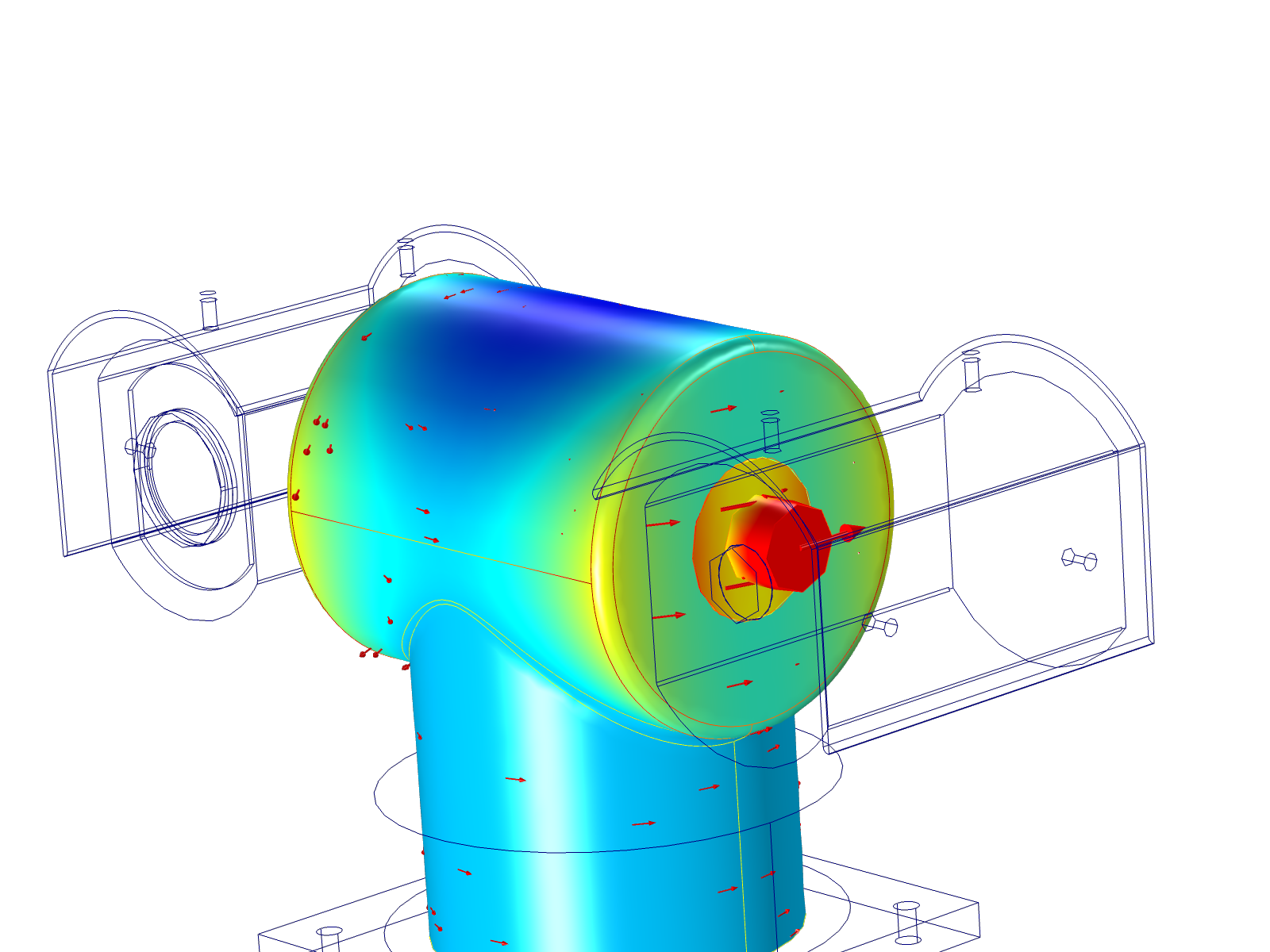
(original design, human-generated)
Once the Parallel Pipes AI-GDP processed the design goals, it ran a joint investigation on the original design. The first component was a mechanical fatigue and endurance test, as seen in “Figure 2”. The second was an optical performance investigation, and because the Custom tier was used, Parallel Pipes’ engineers modified the optical investigation to include various target object examples. This provided more accurate data points with which to evaluate the effectiveness of the optical sources and detectors. As a result, it was determined that optical performance and cost were the primary design variables to improve, in order to obtain the best results. The primary design goals were, in order of importance:
- Reduce production cost
- Enhance performance with object detection up to 40 meters
- Improve durability
- Increase system endurance to stress, corrosion and weather conditions.
Through the project, Parallel Pipes’ engineers advised and supported the company’s team. They only needed to upload their design to the platform, and indicate the type of work the system does, guided by easy-to-use Job Type and Optimization forms. Additionally, the team designated which parts within the design had to remain the same, and which could be optimized with generative design. Setting boundary conditions of allowable ranges for optimization is extremely important, because they determine the outcome nearly as much as the design goals. Additionally, allowable materials to use and acceptable categories of standardized parts were defined, such as LEDs or cameras, were defined. The setup took less than an hour, and after submitting the optimizations to run, the platform allowed the team to view and verify iterations of the optimization process at all times, receiving notifications on the project’s update page.
AI-Guided Optimization
Nowadays almost any engineering assembly, from the simplest widget to more complex products like imaging systems, include standard components as they are cheaper and readily available [2]. For this reason, an AI-guided optimization of optical and robotics systems often needs a combination of standard components and mechanism, and modified geometries done with generative design techniques as a means to obtain the most cost-efficient model.
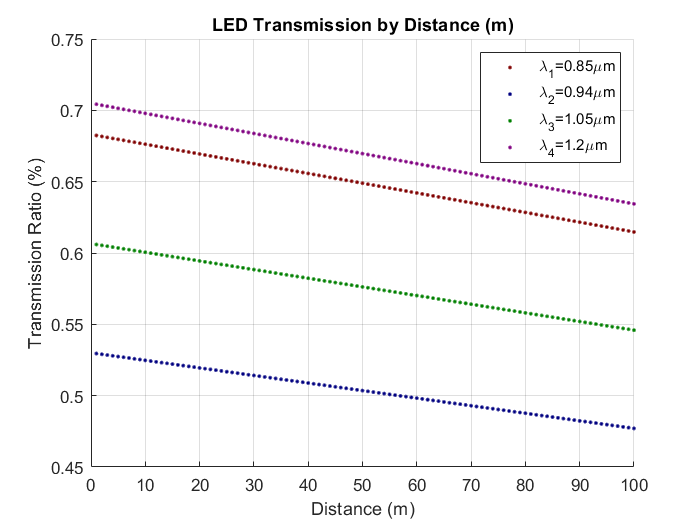
through atmosphere (measured).
Comparing performance of standard components from several reliable manufacturers and distributors gave a good baseline for the AI to evaluate differences (represented in “Figure 3”). Further weights contributing to the overall calculations were cost of components, detection range (distance), and performance measured as the transmission of SWIR and LWIR wavelengths through the atmosphere. However, it was extremely difficult to find components that operate over certain wavelengths identified as “best” by the AI-GDP at a reasonable price, which presented a more complex challenge.
After several iterations as we can see in “Figure 4”, the system performance was optimized in every place that did not cause conflict with the cost-reduction requirement, and preserved if the changes had increased the overall cost-per-unit. One of the biggest improvements the AI-GDP made was the implementation of a set of modular robotics joints that both reduced weight and provided an enhanced flexibility for the design at the best cost. This was not only a cost-related change but a functionality improvement as it also caused much less wear and tear on the system for prolonged use. This was especially convenient for this HSS whose use was expected to be 24/7.
Almost the whole system’s surface was transformed and compacted to meet the design goals. Just as an example of the positive changes, SWIR imaging at night were improved by optimizing the illumination sources, which resulted in an increased effective detection range for target identification up to 40 meters.
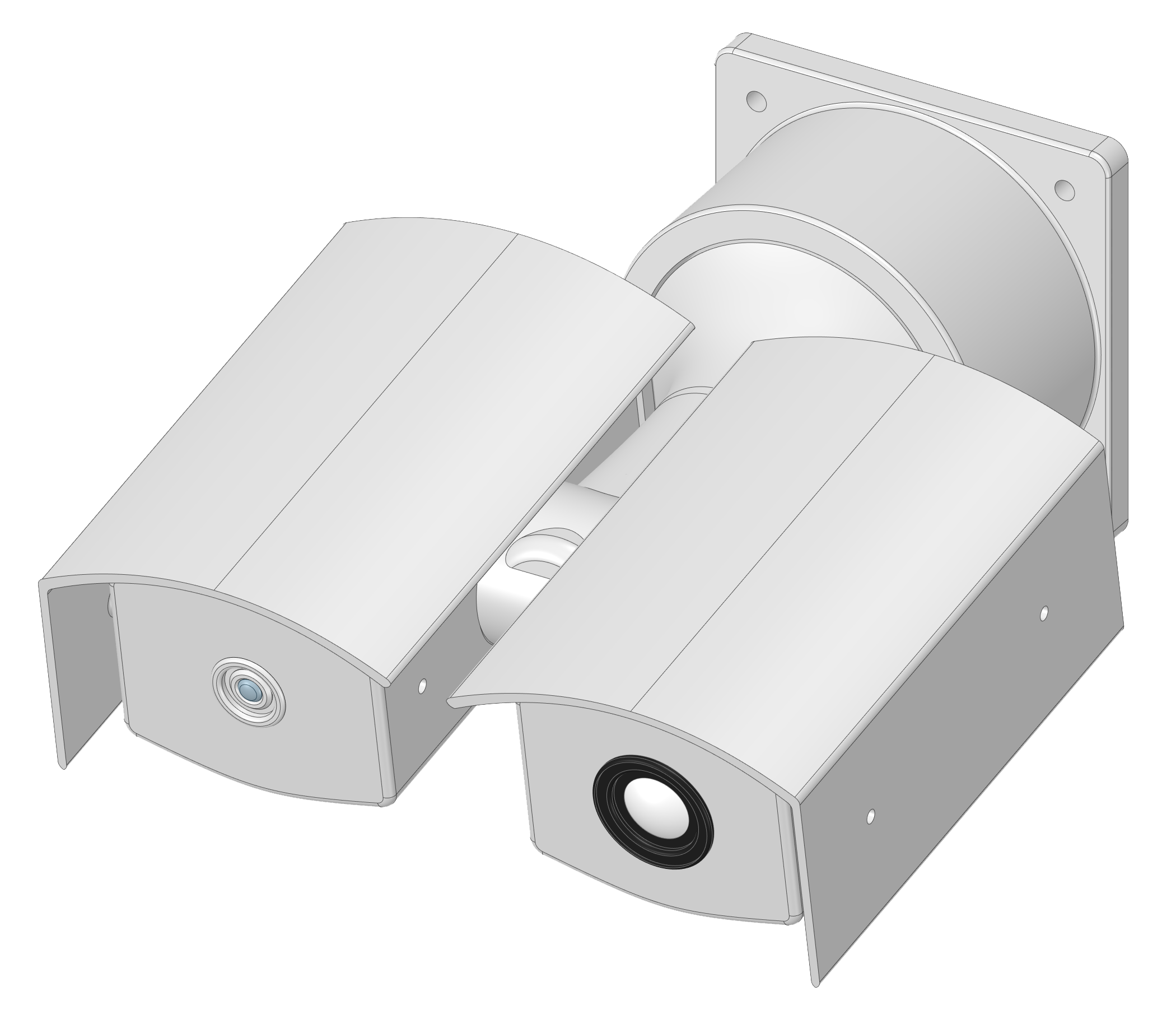
AI-generated model (after 102 iterations).
In addition, the AI-GDP also detected the likeliest source of the noise for the LWIR camera. It suggested as an update to change the camera type and internal cooling, implying that the most probable cause was inadequate cooling. This caused the temperature to rise to problematic levels under certain operating and environmental conditions. The temperature threshold of the LWIR camera used was lower than the temperature reached over prolonged usage. Thus, the optimization of the design goal about optical image quality was achieved through component selection and geometry changes leading to temperature reduction, removing the noise generated by the first design. “Figure 5”, “Figure 6” and " show some of these design changes.
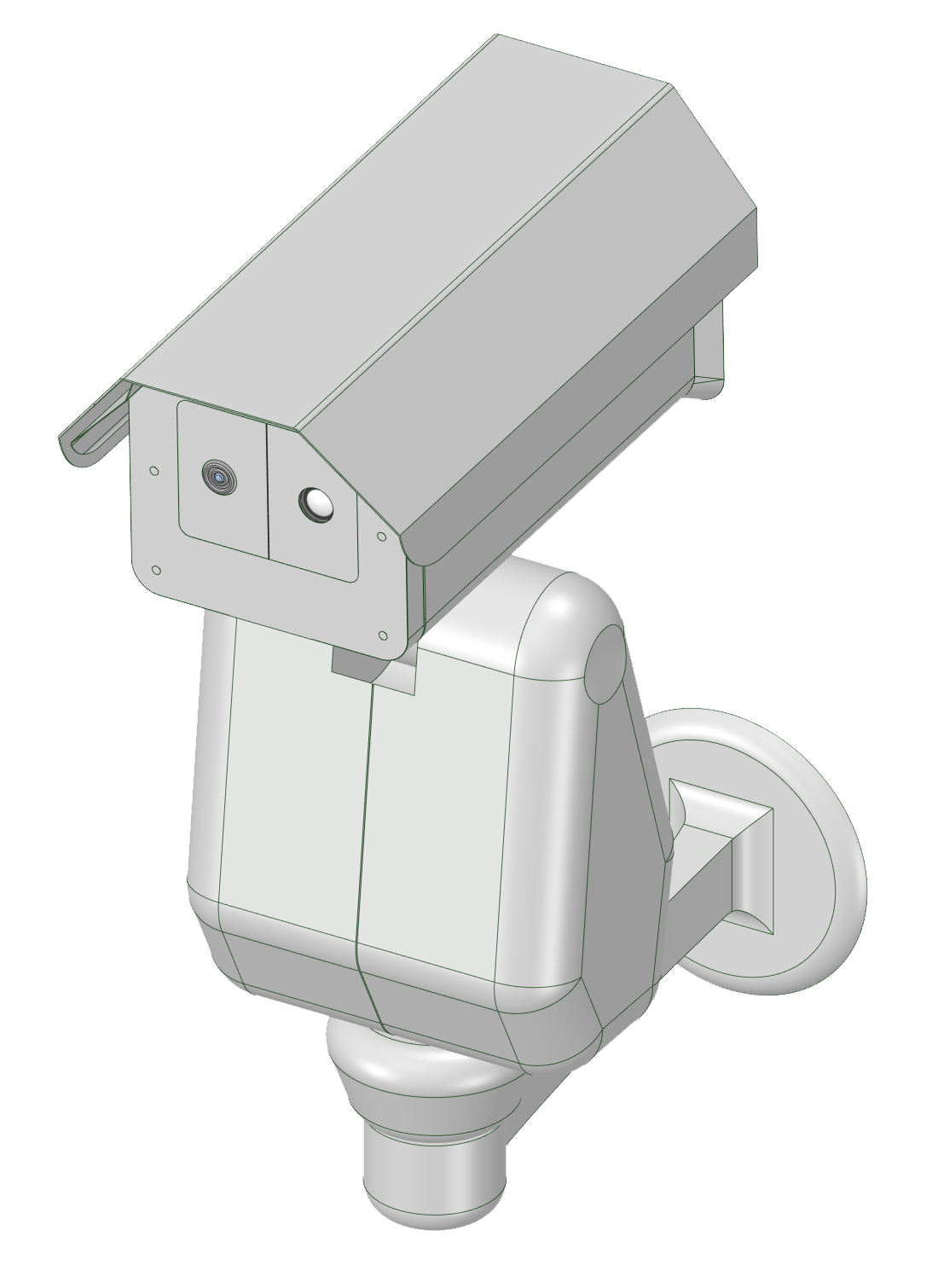
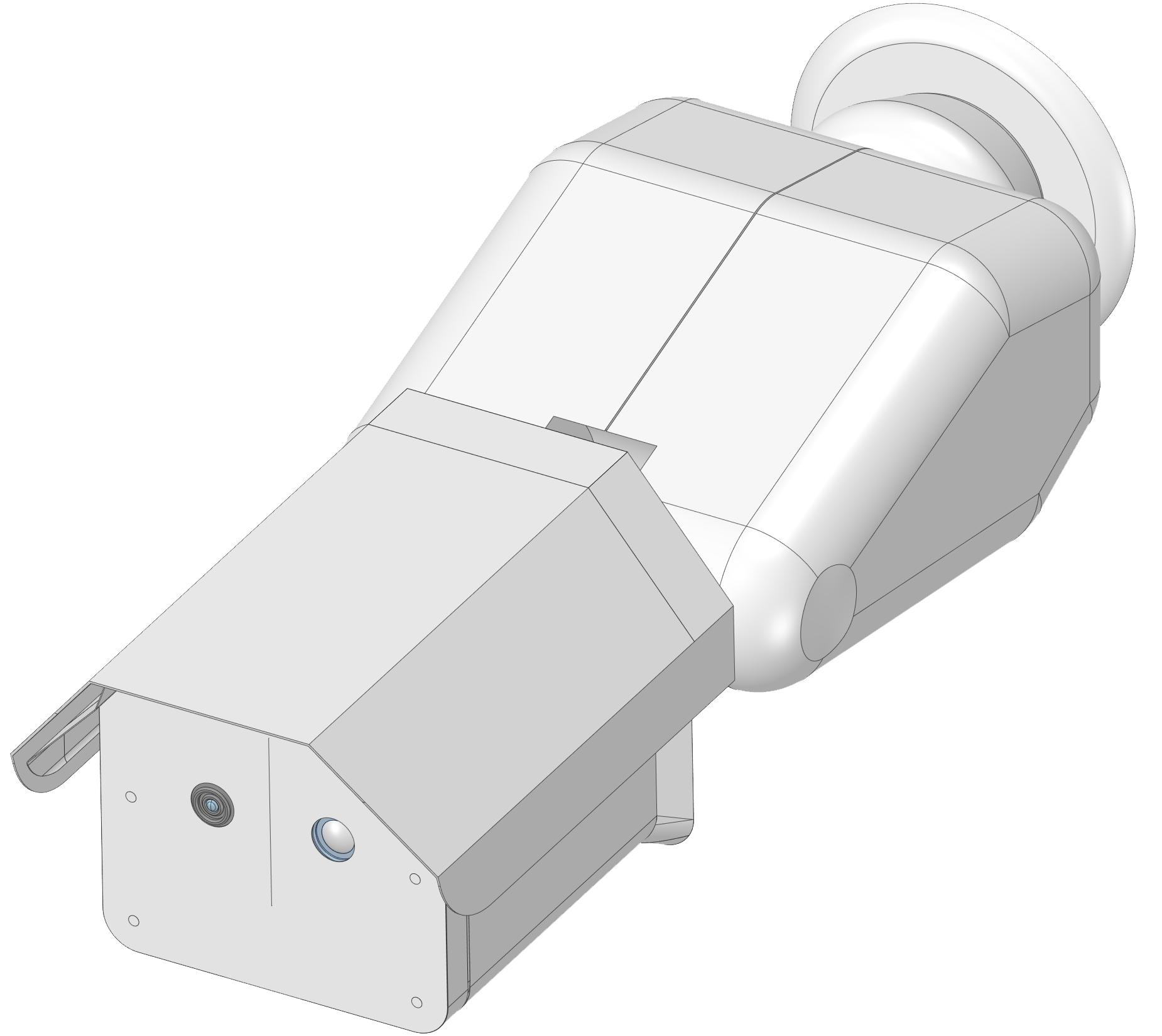
Given the geometric limitations, design goals and boundary conditions, the optimization process was executed in a few cyclically repeating steps. “Figure 7” represents the last test done to the final design on iteration #15,412.
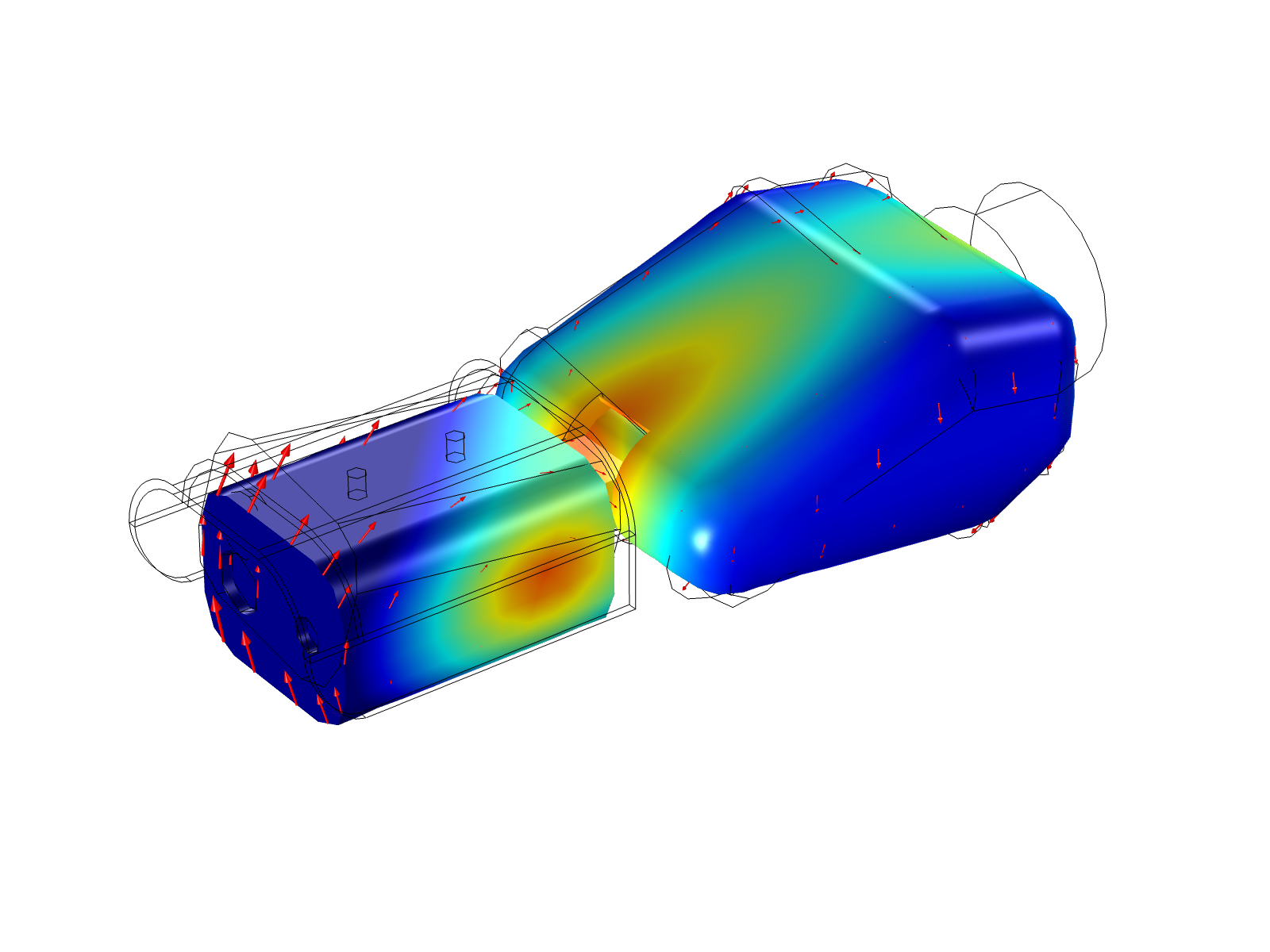
AI-generated model
(after 15,412 iterations).
Throughout the entire process the company was able to check for updates and give feedback to the direction the design was conducted, and request corrections during the course if needed. A good example of how useful this approach can be is the LWIR results; the team was able to verify that this was indeed one source of the problem after a physical test, and allowed the AI to fix the problem proactively.
Using Parallel Pipes the optimization process took only 6 weeks and 3 days, using the Custom Optimization+ tier and some guidance from Parallel Pipes’ engineers.
Results and Conclusion
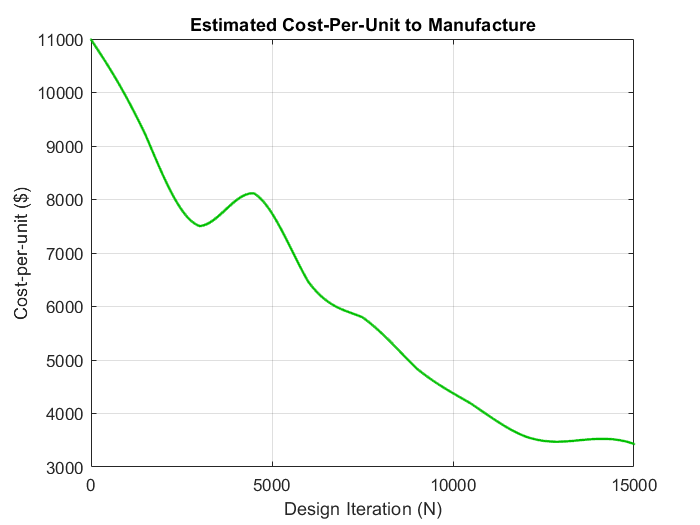
Design iteration (Estimated).
Parallel Pipes’ AI-driven CAE was able to guide and morph the original design submitted by the company’s engineering team, using their own design goals, into a CAD representing the final prototype. Once built, assembled and tested in the physical world, the product will be ready for manufacture: a smarter mass production.
Using this AI-GDP, they were able to reduce estimated development time by more than 3 months, and reduce the manufacturing cost-per-unit by more than 60% compared to the first design, as evidence suggests in “Figure 8”.
As a result of the simulations with the AI-GDP to the prototype, the final design was used by the company to carry out the tests prior to the manufacturing and subsequent launch of the product to the market. This allowed them to clean-up any additional issues by using Parallel Pipes, and thus present a higher quality product to their potential customers.